Features Comparison: Meta’s Robyn vs. Google’s Lightweight
Meta's Robyn and Google's LightweightMMM stand as two open-source advanced MMM platforms. While both tools aim to offer robust modeling capabilities, they differ substantially in philosophy, implementation, and their approach to data optimization.
In this article, we dive into the capabilities and limitations of two leading open-source platforms - Robyn developed by Meta and Google's Lightweight MMM platforms. While both tools promise greater efficiency and precision in marketing analytics using AI, they differ substantially in their technical implementation. We compare key features including model architecture, optimization methods, adstock handling, seasonality, and more. The analysis also covers areas requiring additional expertise when applying these advanced modeling tools.
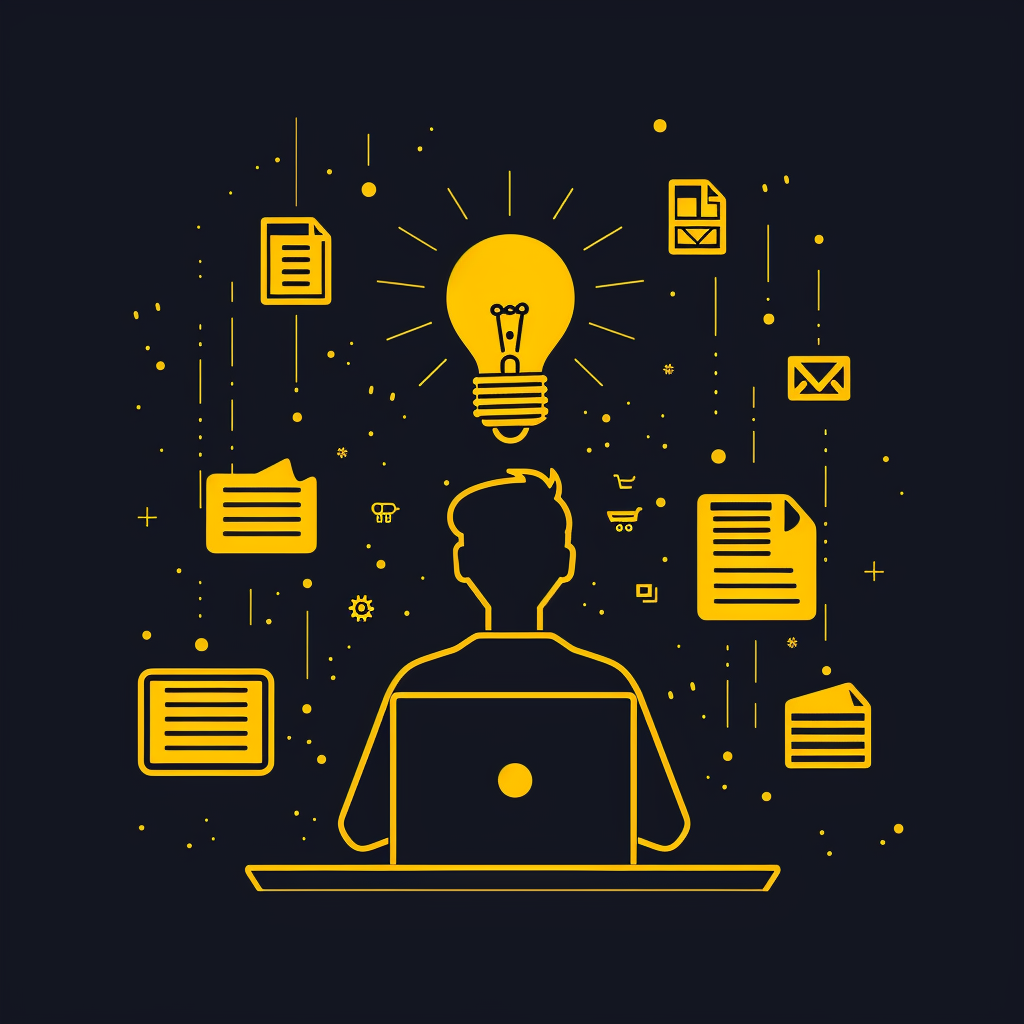
For marketers looking to upgrade their analytics tech stack with AI-powered measurement solutions, understanding the core strengths and tradeoffs of Robyn versus Lightweight provides valuable guidance on selecting the solution that’s best suited for your business needs. The overview helps highlight the most important factors in evaluating how well each platform can address these needs. As MMM enters a new era of AI innovation, the capabilities mapped out in this comparison serve as an important benchmark for the marketing analytics space right now.
Robyn’s Features
Programming Language and Accessibility
Robyn is built using the R programming language, a choice that arises from traditional econometricians and statisticians who predominantly work in R. However, while R is robust for statistical analysis, it is not as widely used as Python, making it less versatile for broader teams that may be more familiar with Python. Robyn’s PM at Meta explored a Python wrapper for Robyn, but opted to hold off until all of Robyn’s major features shipped.
Model Architecture and Run Time
Compared to Google's LightweightMMM, Robyn takes considerably longer to run models. One of Robyn's distinguishing features is its complex model architecture, employing a multi-stage approach. A Ridge regression model is run, followed by the optimization of hyperparameters through Nevergrad's evolutionary algorithm. Additionally, Robyn’s attention to Ad Stock effects is more robust. This complexity results in a longer model run time, but the trade-off is a more nuanced understanding of marketing dynamics.
Advanced Optimization Metrics
For hyperparameter optimization, Robyn goes beyond MAPE (Mean Absolute Percentage Error) and employs its own metric, Decomp.RSSD. It stands for “Decomposition Root Sum of Squared Distance.” Decomp.RSSD minimizes the distance between share of spend and share of effect, to get rid of the extreme disproportionate results based between media channels. This makes the model’s recommendations more plausible, avoiding suggestions of drastic changes in ad spend that may not be feasible or effective in practice.
Detailed Adstock and Saturation Models
Robyn also outshines in its handling of adstocks and saturation effects. It uses a robust variety of models for these factors and dedicates significant computational power to optimize them. In comparison to alternatives like Google's LightweightMMM, Robyn offers a more comprehensive approach by building around 10,000 models to estimate the parameters for adstocks and saturation effects.
Seasonality and Domain Knowledge
Seasonality is another area where Robyn excels. It utilizes Prophet, a Bayesian open-source library, to manage seasonality effectively by accounting for annual seasons, holidays, and other events specific to the country. Moreover, Robyn allows for the inclusion of domain knowledge as a specific feature and optimization target, which is particularly valuable for businesses with specialized market conditions or product offerings.
Decision-making Tools
Robyn provides a rich set of decision-making tools, including waterfall charts and metrics like the share of effect versus the share of spend. These add an extra layer of insight, aiding marketers in comprehending how various channels contribute to overall performance.
Time to Run the Model
Despite its more advanced features, Robyn doesn't require an extended period for project completion compared to other solutions. Generally, defining the business scope, data collection and cleaning, and presenting the results will take approximately 8 to 14 weeks, which is comparable to Google’s LightweightMMM.
Areas of Consideration
Complexity
While Robyn offers robust and comprehensive solutions, there are a few things marketers should be aware of. For companies that don't have the technical expertise, the learning curve can be steep. The complexities associated with its multi-stage modeling and advanced metrics might not be easily grasped by marketing teams without a strong statistical background. The reliance on R could be a constraint for teams more familiar with Python.
Domain Knowledge Requirement
While Robyn provides features for incorporating domain knowledge, these require expertise and manual adjustment. Marketers without a deep understanding of domain-specific intricacies may find it difficult to fully leverage this feature.
Shifting Modeling Windows
Robyn offers a shifting modeling window that allows companies to zoom into more recent data, like the last 3 or 6 months. While this feature is beneficial for incorporating fresh data, it could be problematic for channels with long adstocks or shift effects, leading to significant changes in model parameters that could be misleading.
Google’s Lightweight
Google's LightweightMMM emerges as a modern solution, providing agile and efficient ways for marketers to make data-driven decisions. Here, we dive into the capabilities of this innovative platform and why it's catching the attention of the digital marketing world.
Programming Language
In contrast to Meta's Robyn, which is grounded in R, LightweightMMM is built on Python. Google's embrace of Python makes the LightweightMMM platform more accessible, tapping into a larger pool of developers and data analysts familiar with the language. On the other hand, it may not appeal to traditional Econometricians and Statisticians that prefer R for Marketing Mix Modeling.
Rapid Model Run Time
Speed is one of the standout features of LightweightMMM. This expeditious computation is due to LightweightMMM's configuration, which incorporates the Bayesian model to natively estimate saturation curves and adstock rates. Additionally, its integration with Numpyro and JAX for differentiation offers a 'lightweight' approach, true to the platform's name.
Advanced Optimization
In terms of optimization, LightweightMMM takes a different route than its counterparts. The model emphasizes accuracy, optimizing based on the MAPE (Mean Absolute Percentage Error). This metric provides insights into the model's average day-to-week accuracy. However, to avoid implausible results, Google employs a Bayesian framework. This method sets "priors" that act as safeguards against unlikely outcomes for marketing coefficients. Moreover, Google's media budget optimizer generally suggests only up to a 20% change in spend, ensuring recommendations remain realistic and actionable.
Handling Adstocks and Diminishing Returns
Google's approach to adstocks and diminishing returns is modular and scalable. LightweightMMM provides three main models related to media spend. The default “adstock” model captures the lagged effects of ad spend on sales. For businesses with larger ad budgets that need to account for the diminishing performance as ad spend increases, the "hill_adstock" model is available. Google's method, while simpler than Robyn's multi-model approach, is effective, especially for smaller businesses that may not experience significant saturation effects.
Seasonality
LightweightMMM takes a native approach to seasonality, using a sinusoidal parameter for repeating patterns. The inclusion of an Intercept, trend, and error terms ensures a comprehensive understanding of seasonal variations. Although Google's approach is somewhat different from Robyn's use of the Prophet library, it still addresses the fundamental need for seasonality in modeling, allowing for more strategic marketing decisions during peak periods.
Geo-level Support
Arguably, one of the most distinguishing features of LightweightMMM is its native support for geo regions. When provided with data from multiple geographic areas, the model outputs individual charts for each region, coupled with overarching accuracy and performance metrics. Such granularity not only enhances the model's accuracy but also allows businesses to make more tailored decisions based on geographic nuances.
Domain Knowledge and Adaptability
While LightweightMMM doesn't come with out-of-the-box features for domain knowledge like Robyn, it offers flexibility within its Bayesian framework. Skilled data scientists can modify the platform to accommodate their unique domain knowledge, fine-tuning the model for specific market conditions or business nuances.
Areas of Consideration
Limited Documentation
Google's project is still relatively underdeveloped compared to Meta’s Robyn. It lacks extensive supporting documentation and guides. This could make the initial setup and ongoing management of the platform more challenging for users who are new to MMM.
Less Advanced Optimization
LightweightMMM optimizes based on MAPE alone, which is a measure of accuracy. It doesn't offer advanced metrics like Decomp.RSSD or MAPE.LIFT (for experiments) that Robyn uses. While MAPE is a good measure of average model performance, it may not account for some nuances in spend allocations, potentially making the model less actionable.
Handling Adstock and Saturation
While LightweightMMM offers three main models related to media spend, it doesn't provide as many options for adstock and saturation as Robyn. For businesses with more complex needs, LightweightMMM might not offer the kind of specificity required to accurately model the diminishing returns of advertising spend.
Seasonality Handling: Fixed Assumptions
Although LightweightMMM accounts for seasonality natively, it uses a sinusoidal parameter with a repeating pattern. This approach assumes fixed seasonality effects, which might not be appropriate for all businesses, especially those with variable seasonal trends.
Lack of Domain Knowledge Integration
LightweightMMM doesn’t natively support the integration of domain-specific knowledge in the way that Robyn does. While Bayesian frameworks like LightweightMMM allow for the use of priors, this requires manual intervention and a high level of expertise, making it less accessible for less technical users.
No Feature for Recent Performance Understanding
LightweightMMM doesn’t offer the same flexibility as Robyn in terms of modeling window adjustments to focus on more recent performance data. This can be problematic for businesses with fast-changing environments or those that want to incorporate the most current data, provided that the datasets are large enough, into their models.
Each tool has its distinct advantages and drawbacks. Marketers should consider their specific needs, including the expertise available, the time they can allocate, and the complexity of their marketing channels, before choosing between Robyn and LightweightMMM.